Sequencing, Synthesis, Scale, Software: the New Model for Biology
The 4S model: a new model - or framework - for all biotechnologies.
A model can be broadly defined as a framework, used as an example to follow or imitate.
In genomics, we have something called the read, write, and edit (newly added due to CRISPR) model that has allowed us to examine, add, and even alter genomes.
The creation of this model has ultimately marked a massive transformation in the field of genomics. Only a decade ago, people with a PhD in genetics would often be sequencing a gene. Now, graduate students sequence genomes, and carry out massive functional studies trying to uncover how the order of bases in a DNA affects the traits of a human being.
To extrapolate from the genomics model, we can broadly categorize biotechnologies under what Elliot Hershberg, writer of Century of Biology and someone who I take massive inspiration from, describes as the 4-S model.
The four components of this model are Sequencing, Synthesis, Scale, Software.
Essentially, DNA sequencing and synthesis are being combined to produce large scale data that requires new software to wrangle and analyze it.
But what is the actual purpose of this model?
Helps identify commonalities across a range of projects and scientific papers.
Instead of focusing on the minute details of every scientific paper, we can instead think about what part of the model each project fits in. This simplifies the process of reading otherwise complex papers.
Help us form a broad thesis about the foundational technologies that are powering the modern biotechnology revolution.
We can begin to think about the role that each of these technologies are playing in reshaping our understanding of biology
Sequencing
In order to fully understand this model, let's dissect each part starting with sequencing.
This section refers to DNA sequencing which simply means to uncover the order of nucleotides, specifically the order of four bases: adenine, guanine, cytosine, and thymine. Because of the competition around developing efficient sequencing technologies, the cost to sequence a genome has become increasingly cheaper to the point where it costs less than 1,000 dollars.
The impact of cheap sequencing can not be overlooked. We are now able to explore far more complex relationships because of how affordable it is. For example, we can now begin to examine the relationship between genetic variation and health outcomes. This allows patients to be better with drugs based on their genetic and other personalized data. Furthermore, it allows us to create tools to better monitor our bodies and health.
Specifically, it has been said that the composition of one's gut microbes can be highly predictive of disease outcomes, and can even predict spikes in blood sugar after eating different types of food. Thus, examining our gut microbes, which is just simply the community of microorganisms in our digestive tract, could become essential for understanding how different diets impact our bodies on an individual basis.
With the drop in cost of genome sequencing, projects such as UK Biobank have emerged and have been able to generate whole-genome sequencing (WGS) data for 200,000 individuals, the largest amount of sequencing data in history at that time.
The All of Us program now aims to generate WGS for over a million Americans, really showing how ambitious we can now be. The most dominant force in genomics is Illumina with their development of next-generation sequencing (NGS).
This technology allows us to more quickly and efficiently sequence DNA in comparison to our previous technology in Sanger sequencing. This technology works by using flow cells, which are small devices that contain tiny channels or “lanes” where DNA samples can be placed for sequencing, each of the DNA sequences in the “lanes” can be sequenced simultaneously, greatly improving the speed of genomics. This technology is ultimately what makes modern genomics possible and cheap.
The development of new sequencing technologies does not stop there, companies such as Oxford Nanopore and Pacific Biosciences are both developing long-read sequencing technologies. This makes it possible to analyze complex sequences of the genome that were difficult or impossible to measure with technologies like Illumina.
This moves us closer to a future where an individual’s genome could be sequenced and assembled in an automated fashion.
Synthesis
Moving on, it is now time to discuss synthesis which just refers to DNA synthesis. DNA synthesis is the natural or artificial creation of deoxyribonucleic acid (DNA) molecules. The reason why it is important to efficiently create, artificially or naturally, DNA molecules is because the use cases of DNA are expanding.
DNA is now being constantly used as a substrate, meaning that it is being manipulated or modified to perform specific functions beyond its natural role in encoding genetic information. A central part of the synthesis category is oligonucleotides, commonly called oligos. These short sequences of nucleotides are essential for many of our foundational technologies such as PCR (Polymerase Chain Reaction), the aforementioned Illumina sequencing, and CRISPR for gene editing. Without oligos, these technologies would not work, preventing us from ultimately expanding the use of DNA in a lab environment.
As a result of the importance of oligonucleotides, oligos have become widely accessible, making it possible for them to be designed, ordered, and delivered all in a single day. The importance of oligonucleotides can not be understated.
Oligo synthesis, which is the process of generating multiple oligonucleotide sequences in parallel, is a new tool that has been developed by companies such as Integrated DNA Technologies (IDT) and Agilent. Thanks to large scale oligo synthesis, we are now able to use NGS technologies to measure properties of individual cells called single-cell sequencing.
Single-cell sequencing is extremely important because it allows us to study genetic information of individual cells. Traditional sequencing methods typically analyze a bulk sample of cells and provide an average representation of the genes present. This is slightly problematic because there could be the rare case where a couple of genes strongly affect the regulation of cells while others barely contribute; however, because it is an average you never get to know this and only see how as they group they were around average on their effect of cell regulation.
10X Genomics is a major provider of this single-cell sequencing technology, with an approach designed around using gel beads coated with oligos and containing barcode sequences that can be used to capture and identify individual cells for measurement. This is an example of a breakthrough technology built on top of both sequencing and synthesis.
Another example of the intersection between synthesis and sequencing is in the realm of storage.
Problem:
As a species, we are generating and collecting data at a rate that will soon make efficient physical data storage a hard problem
Solution:
DNA offers an enticing solution because it is the only highly stable nanoscale information storage technology that we know of. Conceptually, an exabyte (one million terabytes) of data could fit in the palm of your hand. Many labs and companies are working on combining large-scale synthesis and sequencing to both reliably store information (synthesis) and retrieve it (sequencing).
Some potential areas of improvement for the synthesis space are as follows:
Increasing sequence length
Increasing the length and size of DNA will mean that we could potentially store more data in DNA
Decreasing cost
Making oligos even cheaper means that it will become easier to continue to develop technologies. One project that is working on this issue is the GP-write project, a collaboration between academic labs and companies such as Twist Bioscience, Ansa Biotechnologies, and DNA Script, which aim to reduce the cost of engineering and testing large genomes in cell lines by more than 1,000-fold, all of this within ten years. Testing large genomes in cell lines refers to the process of evaluating the genetic makeup and functionality of a complete set of genes within cells.
Scale
While the sequencing and synthesis categories both refer to foundational technologies that independently change the space of biology, the scale portion augments the capabilities of sequencing and synthesis, serving as a complementary piece.
The goal of scale is to speed up the processes so that more data can be produced in a faster window of time. The method by which this speed is achieved is through the intersection of the semiconductor and biological revolution.
The semiconductor revolution refers to the development and mass production of computer processors, microcontrollers, and memory chips, enabling the computer revolution and the creation of extremely powerful machines. On the other hand, the biological revolution transitioned from macroscopic descriptions of living organisms to molecular descriptions of cells.
The intersection lies in the fact that with more powerful and eventually cheaper computers, we now have the machinery to run complex and powerful software tools that are being developed in the biotechnology industry. This ultimately allows for faster and better data collection and analysis. A clear example of scale that was already mentioned was sequencing DNA simultaneously in the Illumina sequencing model. Thanks to the semiconductor revolution, we are now able to focus on making an entire system faster.
A clear example of this already happening is from the transition from reporter assays to massively parallel reporter assays (MPRA).
Most experiments consist of perturbing a system and then measuring what occurs. In molecular biology and genetics, perturbing a system involves intentionally inducing changes or disturbances to genes in order to investigate their response and hopefully understand their function.
Going back to the reporter assays, these are perfect examples of this experimental model. Reporter assays are often used in molecular biology to figure out what regulatory sequences of DNA, which are just specific segments of DNA that control the activity of genes (on or off), are necessary to turn a gene on (meaning that it is actually functioning and producing proteins) and off.
The main premise is to construct a DNA strand that has both the regulatory element in question (the one responsible for turning the gene on or off) and a gene that can be easily detected when it is expressed (when the gene is on and producing proteins). The end goal of a reporter assay is to hopefully understand the function of a specific sequence of DNA. Historically, the core measurement tool would often be a reporter protein such as green fluorescent protein (GFP). Reporter proteins are just proteins that can be easily detected.
The major shift is that instead of measuring using a reporter protein we can now synthesize or mix them in a pool of oligos. This allows us to test a massive number of different sequences in parallel, hence the creation of the MPRA.
The results of an MPRA are then measured using sequencing. This is accomplished by taking the ratio RNA to DNA of a specific barcode in the oligo pool. If a sequence drives RNA expression, we should see far more RNA copies of the barcode than the original DNA barcode because the DNA activated the genes and told them to produce RNA.
This is a really important change because it means that we can explore more DNA strands quicker. This is especially huge for human geneticists who have been trying to understand how DNA differences lead to differences in traits.
One of the biggest challenges though is that most of your complex traits or traits that are influenced by multiple genetic factors (height, intelligence, or susceptibility to disease) are not actually influenced by the parts of your DNA that affect the DNA sequence in genes.
Genes are specific segments of DNA that provide instructions for the production of proteins or functional RNA molecules, the sequence in these genes ultimately influencing what proteins are produced which in turn influence your traits. However, many of our complex traits are influenced by genetic variants that do not affect the sequence of DNA inside genes, meaning that it becomes challenging to understand and explain how they influence these traits. It is not as straightforward as them affecting the sequence inside genes because they might actually be impacting gene regulation, protein interactions, or other complex molecular processes. Now that we have the technology to efficiently test countless strands of DNA, testing a huge variety of strands is not nearly as expensive.
To extrapolate from this one example, if you can first find a way to effectively use sequencing and synthesis approaches to tackle a problem, we can then begin to scale the experimental throughput. For any tool that can fit into this paradigm, you can go from a dozen to thousands of measurements.
Software
Our final section of the model is software. Similar to scale, this section also serves as a complementary piece to our core synthesis and sequencing technologies.
The goal of software is to compile and analyze the huge amount of data produced by both DNA sequencing and synthesis. While collecting data is important, the data means nothing without proper analysis and dissecting the key points.
Bioinformatics is literally an entire scientific discipline focused on developing software to better analyze data. Similar to scale, the better the software, the more efficient our DNA sequencing and synthesis technologies. Without proper software, sequencing a genome would not be possible.
The effectiveness of software in making data both readable and sensible has led to a concept called Software 2.0 as described by Andrej Karpathy, Director of AI at Tesla. The essential premise is that previously we used complex math and physics equations to help us understand the laws of our universe; however, we can now use an immense amount of data to understand the laws that define our universe.
In other words, instead of attempting to write down the rules of a program that would get us the solution, we work backwards by collecting large volumes of data that represent the solution, allowing us to understand the rules of the program.
A perfect example of Software 2.0 is machine learning. Recently, machine learning was used to effectively solve the protein structure prediction problem.
Similarly to translating languages, there is no simple equation to understand how amino acids fold into 3D machines; however, it is possible to learn how using massive amounts of data and enormous models.
Another use of software is as a platform for design and discovery.
Problem:
There is no dedicated platform for biological design and discovery, making it difficult to compare experiments in the space as everyone uses different methods.
Solution:
One approach has been to develop pure software platforms for scientists. In genomics, genome browsers have become the de facto representation of genetic information. A huge benefit of a dedicated genome browser is that they help eliminate waste and inefficiency. With common infrastructure, individual groups and companies don’t have to reinvent the wheel and build the same tools that everybody else is using, there is now a standard, making it easier to share and edit work.
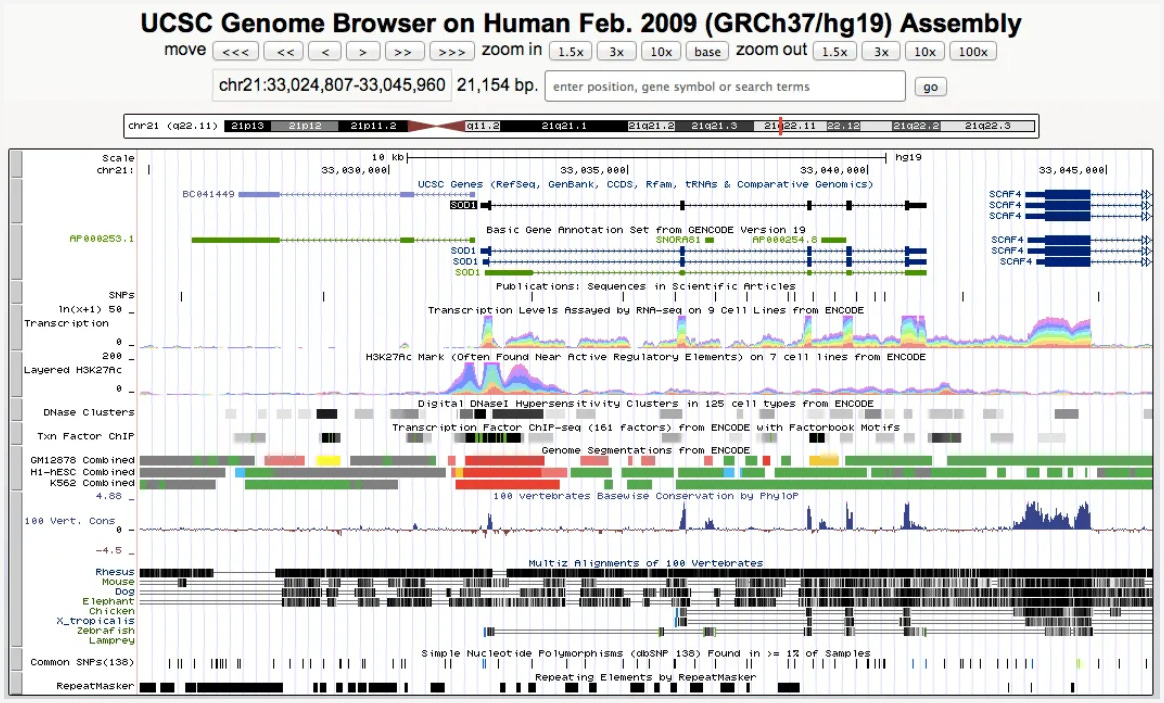
This new biological revolution will constantly require new platforms spanning the entire spectrum from pure software all the way to model-driven labs. This makes for a fantastic opportunity for talented engineers to use their expertise to create an abundant future in the physical world. Who knows how the next software can affect how we approach biology and science as a whole.
Sources:
https://centuryofbio.com/p/4-s-model
https://centuryofbio.com/p/whats-different-part-one-sequencing
https://centuryofbio.com/p/whats-different-part-two-synthesis
https://centuryofbio.com/p/whats-different-part-three-scale
https://centuryofbio.com/p/whats-different-part-four-software