Exploring Sickle Cell Disease
Brandon Le's work in sickle cell disease, exploring its genetic pathways and variants
Sickle cell disease is a genetic disorder where your red blood cells become sticky and turn into a sickle shape. As a result, these cells will physically block your blood vessels, causing them to block arteries, veins, and capillaries, completely destroying blood vessels. This disease set the foundation for my discussion with Brandon Le, a graduate student at Duke University currently working at Dr. Allison Ashley-Koch’s lab.
Brandon Le is largely interested in genetics and genomics, specifically statistical genetics and genetic epidemiology. Brandon decided to work with Dr. Allison Ashley-Koch mainly due to the strong community and exciting project. He felt that the lab was full of passionate researchers who were eager to contribute. Furthermore, Brandon was intrigued by the data for the project because there were so many potential avenues that he could explore.
Background
In the first segment of our discussion, Brandon provided some important background on sickle cell disease, which was the subject of his current project. Sickle cell disease is what we call a mendelian disorder, which means that a single genetic mutation will cause sickle cell disease. The genetic mutation that causes this is a gene called beta-globin, which is part of the hemoglobin molecule. As previously described, when this genetic mutation occurs, your red blood cells will turn into a sickle shape, which in turn causes sickle cell disease and the earlier mentioned effects.
Sickle cell disease is usually triggered under hypoxic, low oxygen conditions. Hypoxic conditions occur more often than you think. While you may normally think of low oxygen situations as times where you are at high altitudes, these situations may occur during cold weather or intense physical labor such as running or going to the gym. Essentially any time that you are breathing really hard is because your body is trying to increase your breathing rate so your cells and tissues can get more oxygen. For healthy people, we don't have to worry about when we go to the gym or for a run because we don't have sickle cell disease; however, for people with that disease, even going exercising can cause sickle cell disease problems such as:
Pain crises
This is characterized by a very acute and sudden rapid onset occurrences of pain throughout the entire body.
Lightheadedness and chance of fainting
Essentially, hypoxic conditions are very dangerous for people with sickle cell disease because if they fail to cool down or stop to make sure that their bodies return to a normoxic condition, they can easily pass out and endure further and more serious sickle cell disease complications.
One very interesting thing that the lab found was that people that only receive one copy of this beta-globin mutation actually exhibit resistance to malaria and what we call sickle cell trait. This point links nicely to the fact that this genetic mutation primarily affects people who have African or Indian genetic ancestry, the two regions that are known to be endemic to malaria. The lab used this fact to hypothesize that the only reason that this mutated gene has evolved and persisted in the first place is to provide malaria resistance. Essentially, people who had this trait survived in malaria endemic places such as India and Sub Saharan Africa and continued to pass this trait on.
People with sickle cell trait face similar problems to people with sickle cell disease but at a reduced intensity. They still produce enough normal hemoglobin that they do not need to worry about being under hypoxic conditions, but there are still certain complications that can come up. For example, these people would still have sickled red blood cells that can affect the kidneys and degrade kidney function. However, most of these problems occur later in their lives in comparison to sickle cell disease patients who might experience kidney failure around the age of 30-40.
Project
While we know that a specific mutation in the beta-globin gene causes sickle cell disease, Brandon’s project aimed to find the specific mutations that would most impact the development of sickle cell disease. Using this, the lab would be able to engineer a vaccine that can fix this mutation and potentially cure sickle cell disease.
Brandon’s team looked at two specific metric throughout their studies:
Estimated Glomerular Filtration Rate(eGFR):
How quickly your blood is filtered by your kidneys.
Proteinuria:
The protein content of your urine. In healthy people, you expect that there's essentially no protein content in your urine and any kind of deviations from this is a sign that you may have some kind of kidney damage.
The reasoning behind choosing these two measurements is that the lab found that these two metrics are related to either early or mortality in sickle cell disease patients or a lower quality of life. Essentially, these two renal outcomes are good predictors of how well or how poorly a person with sickle cell disease might progress throughout their life.
The lab used data provided by NHLBI (National Heart Lung and Blood Institute), specifically from the TOPMed program. TOPMed tries to sequence the genomes of as many people as possible so that it can be publicly available to analyze for researches such as Brandon. Brandon is specifically looking at a bunch of sickle cell disease cohorts which just has data from patients with sickle cell disease.
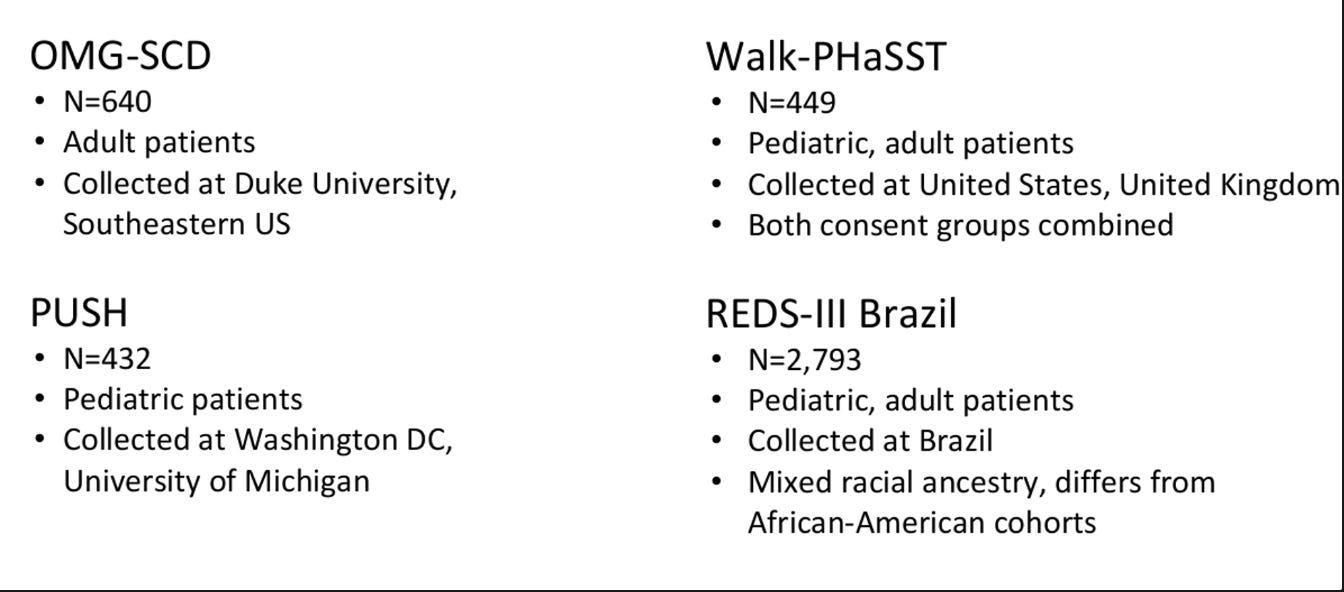
Brandon also used an analysis tool called BioData Catalyst, which is another initiative by NHLBI. This is a cloud computing platform that allows Brandon to analyze huge datasets.
With these datasets and analysis tools, Brandon was able to make numerous comparisons such as do lower eGFR scores correlate to a lower quality of life or a shorter lifespan. The advantage of having so many cohorts is the variety of data that Brandon was presented with. Each cohort represented a specific group with the OMG SCD cohort mainly consisting of just adults and the PUSH cohort being mainly patients under 18. Using these two specific groups, Brandon could compare how sickle cell disease affects patients of different ages. Essentially, the possibilities are endless.
For his project, Brandon performed a genome wide association study (gwas). This process involved taking the genetic data after sequencing all of your patients and then asking for every single base pair in your genome or SNP (single nucleotide polymorphism), what would a mutation in that base pair cause. For example, does a mutation that causes a change from A to T result in a change in the eGFR score. The lab then does this repeatedly across all of the different mutations (every possible base pair change) within each chromosome.
From this analysis, Brandon was able to create numerous graphs that tell them how strongly a specific mutation is related to a particular outcome. While this graph may look confusing, let’s dissect it piece by piece:
Each point on the graph represents a single mutation or SNIP.
The x-axis represents physically where each mutations is located.
The y-axis measures how strongly associated the mutation is with a particular outcome.
The lab established cutoffs (each of the horizontal lines) that determines how statistically significant a mutation is. The cutoffs essentially differentiate mutations that by chance cause a disease or disorder from genes that are guaranteed to affect you, getting rid of any false positives. The blue line represents normal significance or 5 * 10^-5. These mutations are significant but there is still the possibility that they by chance caused something. Maybe you didn't have enough people in your study or your data quality isn't that high. However, on the middle the graph, the lab included an extra red cutoff value (the other ones don’t have it because no point reached that high, which represents points that are 5 * 10^-8. The red line is what we call genome-wide significance because it is the accepted threshold for statistical genetics or marks the place where a mutation should be further studied.
What the lab was essentially looking for in these graphs were really strong signals, for example, all the people with said mutation have a really low eGFR score and all the people without it have a really high eGFR score. In identifying this trend, you can assume the probability of that happening by chance is essentially zero. This creates an obvious signal that would warrant further analysis. In summary, these cutoffs essentially allow the lab to focus on a specific few mutations, narrowing down what they need to further analyze.
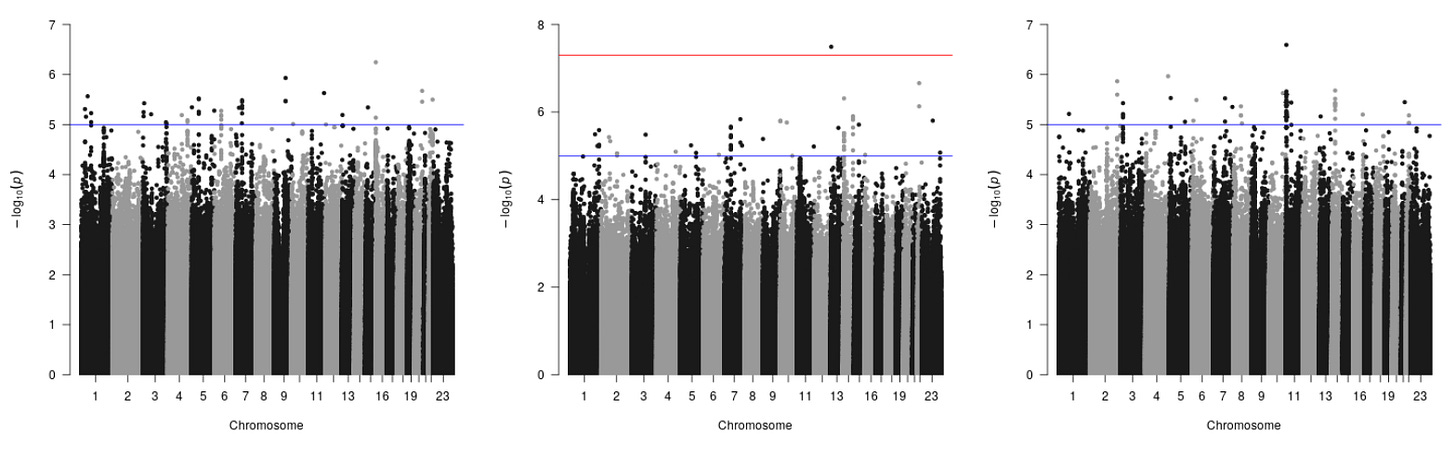
Challenges
BioData Catalyst
Since this was a relatively new platform, developed only two or three years ago, Brandon was one of the first users on the platform so he spent a lot of time debugging all of the tools.
Data
A big problem was ensuring that the data was accurate and that there were no errors during the sequencing process. This is a really important issue to tackle, in biology, there is currently a thing called a reproducibility crisis in biology because it is very easy to make a hypothesis, obtain some data that supports your claim and then make your conclusion/association while in reality your results could have been based on chance or some error in your data. If your end goal is to cure a disease, you need to confirm your results in other way, maybe by seeing if fixing the mutation actually causes the disease to go away (which inversely proves that the mutation does cause the disease).
How can we filter our data to only include the most meaningful figures?
How do we deal with faulty data? One of the patients had an eGFR score of 8,000, which is physically impossible. Does the lab go back to the sequencing facility to ask for a new dataset or do they just eliminate that and continue with a smaller sample size.
Data Management
How do they store the large amount of sequencing data. This issue was actually solved by NHLBI and all the other NIH institutes where they developed a few different tools for researchers like Brandon to actually store this data on the aforementioned TOPMed program. This service is really convenient because the NIH can just manage these datasets and it allows for easier sharing. Honestly, it is really cool how all of these NIH institutes are coming together to fix pressing issues in the scientific space.
Other Work
Besides his main project, Brandon has been working on a few other projects.
One extremely interesting project is with mitochondrial data. This is a really new type of study, only within the paste five years have people actually started to look into this. Some background on mitochondria, the mitochondria is of course the powerhouse of the cell. There are actually between 20-200 mitochondria per cell, it really depends on how much energy a cell needs. For example, something like your heart, arm, or leg muscles will have more mitochondria (100-200) because they required a lot of energy; however, fat or liver cells have way less because they expend less energy.
Mitochondria have their own genomes, cell walls, and even internal cell membranes. Brandon was wondering if there is an association with sickle cell disease outcome within the mitochondrial genomes. In other words, could a mutation in the mitochondrial genome be associated with sickle cell disease in any way. Brandon was also looking to see if there is a difference between people in different haplogroups. A halpogroup is just a fancy word for a group or class of mitochondria. People of different ancestry have different haplogroups. What Brandon can do is split a population based on their haplogroups (L, A, and B) and see if there is a difference in mitochondrial mutations or sickle cell disease outcomes.
With these questions in mind, Brandon used the mitochondrial data from the same four cohorts that were shown before. This happened because in the process of sequencing the entire genomes of the people you're looking at, you tend to pick up on other other genomes such as mitochondrial genomes. What happens is when you take a cell sample or tissue sample from new people, you blend it up, and you purify the DNA inside of it. Well, there's not just human DNA, there's other DNA such as mitochondrial DNA. They then preceded to plot on a graph how many mitochondria per cell (copy number) do people have on average. The majority people had somewhere between 100-120 copies of mitochondria per cell. Brandon and his team were interested in why some people had an absurd amount such as 500 mitochondria per cell on average.
From this project, the team concluded that people with a higher average copy number per cell happen to be associated with certain disease. Brandon is making the hypothesis that maybe one of the associated disease is sickle cell disease.
The last project that Brandon mentioned that he was involved in was a heteroplasmy study. When mitochondria divide, they divide just like bacteria do. They do binary fission, meaning that they grow until they eventually split in half. For mitochondria, they do not undergo the process of recombination; instead, they always split in half and directly copy their entire genome from start to finish, preferable no mutations. Thus, there should be direct copies from one generation to the next, meaning that our mitochondria should be conserved. Heteroplasmy is what happens when one copy is different from the other for some reason. Those kinds of mutations are what Brandon was trying to look at. Specifically, Brandon is trying to see if these mutations are related to genetic human diseases such as cancer and potentially sickle cell disease.
For this project, Brandon is asking the question: within a single person, how many different copies of how many different mitochondrial genomes do we see? He then plotted his data on a graph. For people who have who are in the zero column, that means that all of their mitochondria have the same genomes, no mutations. For people in column one, they have at least one outlying mitochondria and it has at least one mutation and so on. As mentioned before, some mutations do not actually have any effect on us; however, with mitochondria, if you see a mutation, it has been shown that it usually suggests that a problem has occurred, or that it will cause problem. If you try to change the mitochondrial genome, scientists have found that the mitochondria is really sensitive to changes and that it just doesn't work as well. In contrast, if you give a random mutation to a human genome, it is probably going to still keep working. Brandon found that in the OMG-SCD sickle cell disease cohort, there are lots of people that have at least one heteroplasmy, which raises questions if there actually is an association between mutation in the mitochondria and sickle cell disease.
Overall, my interview with Brandon showcased the amount of things that you can study with a dataset. Each dataset provides you with a ton of possible avenues and it entirely matters on your enthusiasm to come up with a project idea. Furthermore, our discussion really highlighted the immense potential of the genomic and genetics field. With the completion of the human genome project in 2003, we have the ability to ask a lot more complex scientific questions. Hopefully, with the continued advancement of both of these fields, we can continue to cure genetic disease such as cancer and sickle cell disease, moving us closer to a future where genetic diseases are a thing of the past.